Dependable Clustering Method of Flight Trajectory in Terminal Area Based on Resampling
-
摘要: 为了准确掌握终端区空中交通流复杂多变的空间分布特征,有效评估、优化进离场程序,基于重采样技术研究了终端区三维真实飞行轨迹的聚类问题,提出了一种计算速度快、可扩展性强、可信度高的聚类方法.首先,结合重采样和主成分分析方法,将高维轨迹数据在保留飞行特征的前提下映射到低维空间;其次,基于MeanShift方法建立飞行轨迹聚类分析与异常轨迹提取模型;最后,利用终端区的真实飞行轨迹数据进行实例验证,并分析模型中各个参数对聚类结果的影响.研究结果表明:该方法耗时0.004 s得到累计贡献率为96.16%的主成分,较好地逼近原始飞行轨迹数据;相较于层次聚类法,本文方法得到的飞行轨迹聚类结果具有更高的可信度,能够准确对应机场标准进场航线设置,并将相似度较低的飞行轨迹提取为异常轨迹.Abstract: To master the complex and changeable spatial distribution characteristics of air traffic flow in a terminal area accurately, and to evaluate and optimize arrival and departure procedures effectively, the cluster problem of 3D real flight trajectories in a terminal area was addressed using a resampling technique. A clustering method that has high computation speed, good expandability, and strong dependability was also proposed. First, based on resampling and principal component analysis method, projection of high-dimensional trajectory data to low dimension was implemented based on the premise of maintaining flight characteristics. Then, flight trajectory cluster analysis and outlier trajectory detection models were presented based on the MeanShift method. Finally, the proposed method was verified using real flight trajectory data of terminal areas in order to analyse the effect of every parameter on cluster results. Experimental results show that principal components having a 96.16% accumulative contribution rate can be obtained in 0.004 s. Flight trajectory data can be well approximated by the principal components. Compared with the hierarchical clustering method, the proposed method can obtain more dependable flight trajectory clustering results which correspond to the standard arrival routes. Low similarity trajectories were detected as outliers.
-
王超,韩邦村,王飞. 基于轨迹谱聚类的终端区盛行交通流识别方法[J]. 西南交通大学学报,2014,49(3): 546-552. WANG Chao, HAN Bangcun, WANG Fei. Identification of prevalent air traffic flow in terminal airspace based on trajectory spectral clustering[J]. Journal of Southwest Jiaotong University, 2014, 49(3): 546-552. ATEV S, MILLER G, PAPANIKOLOPOULOS N P. Clustering of vehicle trajectories[J]. Intelligent Transportation Systems IEEE Transactions, 2010, 11(3): 647-657. FERREIRA N, KLOSOWSKI J T, SCHEIDEGGER C E, et al. Vector field k-means: clustering trajectories by fitting multiple vector fields[J]. Computer Graphics Forum, 2013, 32(3): 201-210. HU Weiming, XIE Dan, FU Zhouyu, et al. Semantic-based surveillance video retrieval[J]. IEEE Transactions on Image Processing, 2007, 16(4): 1168-1181. KALAYEH M M, MUSSMANN S, PETRAKOVA A, et al. Understanding trajectory behavior: a motion pattern approach[J]. Crcv.Uc.Edu, 2015: 1-14. LEE J, HAN J W, WANG K. Trajectory clustering: a partition and group framework[C]//The 5th ACM SIGKDD International Conference on Knowledge and Data Mining. SanDiego:[s. n. ], 2007: 593-604. LEE J, HAN J, LI X, et al. Traclass: trajectory classification using hierarchical region-based and trajectory-based clustering[C]//The 34th International Conference on Very Large Data Bases. Auckland.: VLDB, 2008: 1081-1094. LEE J, HAN J, LI X. Trajectory outlier detection: a partition-and-detect framework[C]//IEEE 24th International Conference on Data Engineering. Cancun: IEEE, 2008: 140-149. 王超,徐肖豪,王飞. 基于航迹聚类的终端区进场程序管制适用性分析[J]. 南京航空航天大学学报,2013,45(1): 130-139. WANG Chao, XU Xiaohao, WANG Fei. ATC serviceability analysis of terminal arrival procedures using trajectory clustering[J]. Journal of Nanjing University of Aeronautics and Astronautics, 2013, 45(1): 130-139. 王超,王明明,王飞. 基于改进的模糊C-Means航迹聚类方法研究[J]. 中国民航大学学报,2013,31(3): 14-18. WANG Chao, WANG Mingming, WANG Fei. Trajectory clustering method research based on improved fuzzy C-Means[J]. Journal of Civil Aviation University of China, 2013, 31(3): 14-18. 王超. 飞行程序运行评估的理论方法及仿真应用研究[D]. 南京:南京航空航天大学,2012. 董菁,张毅,张佐,等. 基于主成分分析法的城市交通路口相关性分析[J]. 西南交通大学学报,2003,38(6): 619-622. DONG Jing, ZHANG Yi, ZHANG Zuo et al. Principal component analysis of dependency of urban intersections[J]. Journal of Southwest Jiaotong University, 2003, 38(6): 619-622. FUKUNAGA K, HOSTETLER L D. The estimation of the gradient of a density function with applications in pattern recognition[J]. IEEE Transactions on Information Theory, 1975, 21(1): 32-40. CHENG Yi. Mean shift, mode seeking, and clustering[J]. IEEE Transactions on Pattern Analysis and Machine Intelligence, 1995, 17(8): 790-799. NIKUNJ O. Flight tracks, Northern California Tracon.[2013-03-1]. https://c3.nasa.gov/dashlink/resources /132. 期刊类型引用(16)
1. 王志森,张召悦,冯朝辉,崔哲. 终端区飞行轨迹聚类分析及异常轨迹识别. 科学技术与工程. 2022(09): 3807-3814 . 百度学术
2. 姚学成,胡明华,袁立罡,陈海燕,刘振亚. 对流天气下基于聚类算法的终端区交通流分析. 航空计算技术. 2022(03): 42-46 . 百度学术
3. 郁舒昊,周辉,叶春杨,王太正. SDFA:基于多特征融合的船舶轨迹聚类方法研究. 计算机科学. 2022(S1): 256-260 . 百度学术
4. 杨璐,李印凤,傅子涛,肖淑敏,魏秀杰. 基于自适应谱聚类的终端区进场轨迹识别. 指挥信息系统与技术. 2022(04): 63-68 . 百度学术
5. 李楠,强懿耕,樊瑞. 基于轨迹压缩的航空器飞行轨迹聚类研究. 重庆交通大学学报(自然科学版). 2021(01): 1-6 . 百度学术
6. 李超群,李善梅,马维宇,张程. 基于轨迹聚类的空中交通流自动识别方法研究. 计算机仿真. 2021(10): 73-77 . 百度学术
7. 纪新雨,初建宇,李印凤,傅子涛,李萌. 基于改进CURE算法的终端区航迹聚类. 指挥信息系统与技术. 2021(06): 63-67 . 百度学术
8. 赵元棣,田英杰,吴佳馨. 航空器飞行轨迹相似性度量及聚类分析. 中国科技论文. 2020(02): 249-254 . 百度学术
9. 赵元棣,付云峰,吴佳馨. 航空器飞行轨迹表示方法及其应用. 科学技术与工程. 2020(12): 5000-5004 . 百度学术
10. 李楠,孙瑜,强懿耕. 机动区主流滑行路径异常轨迹检测. 计算机仿真. 2020(07): 23-27 . 百度学术
11. 赵立新. 差异性采样下的流数据聚类算法分析. 计算机产品与流通. 2019(01): 167-168 . 百度学术
12. 孙石磊,王超,赵元棣. 基于轮廓系数的参数无关空中交通轨迹聚类方法. 计算机应用. 2019(11): 3293-3297 . 百度学术
13. 李树仁,卢朝阳,任广建. 基于改进谱聚类的终端区航空器飞行轨迹分析. 武汉理工大学学报(交通科学与工程版). 2019(06): 1130-1134 . 百度学术
14. 李楠,强懿耕,孙瑜,邓人博. 终端区航空器异常轨迹识别研究. 中国安全科学学报. 2018(11): 21-27 . 百度学术
15. 戴福青,庞笔照,任治,赵元棣. 面向管型航路运行的交叉点拥挤风险评估. 武汉理工大学学报. 2018(02): 42-49 . 百度学术
16. 李楠,强懿耕,邓人博. 基于能量高度的终端区异常轨迹识别研究. 武汉理工大学学报. 2018(10): 38-43 . 百度学术
其他类型引用(21)
-
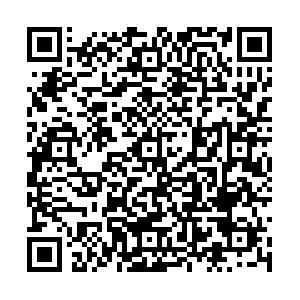
计量
- 文章访问数: 670
- HTML全文浏览量: 94
- PDF下载量: 207
- 被引次数: 37