小样本条件下可变作用代表值的贝叶斯推断方法
doi: 10.3969/j.issn.0258-2724.2014.06.010
Bayesian Methods for Inferring Representative Values of Variable Actions in Small Sample Situations
-
摘要: 为克服工程实际中测试数据不充足的条件下,采用经典统计学方法推断可变作用的代表值时,由统计不确定性导致推断结果偏于冒进的缺点,基于极小值I型分布分位值的线性回归推断方法,提出了小样本条件下可变作用代表值的线性回归推断方法,并以此作为检验其他推断方法精度的基准方法;根据贝叶斯理论,利用分布参数的Jeffreys无信息先验分布,提出可变作用标准值和频遇值的贝叶斯推断方法. 应用研究结果表明:贝叶斯推断方法较线性回归推断方法简便且应用范围广,在标准差已知的情况,可给出更优的推断结果;无参数信息时,对于标准值和频遇值的保证率不低于0.90的情况,贝叶斯推断方法均具有较好的精度;相对于其他可信水平,可信水平0.75时推断结果更接近真值,因此,建议取可信水平为0.75.Abstract: There is a need sometimes in engineering to infer the representative values of variable actions in cases when the test data is not sufficient, but the classical statistics methods do not take into account of the succedent influences of statistical uncertainty, and the inferred results are always on the aggressive side. In order to overcome the above shortcomings, applying the current linear regression estimation for inferring the fractiles of type I minimum distribution, the linear regression estimation of representative values of variable actions is proposed as a reference method for the precision inspection of other inference methods. According to Bayesian theory, applying Jeffreys non-informative prior distribution, a Bayesian method for inferring characteristic and frequent values of variable actions is put forward. The results show that the Bayesian inference method is more convenient than the liner regression estimation, and easy to use in a broader context. The method can give more advantageous results when standard deviation is known. If no parameter information is available, the Bayesian inference method has better precision when the guarantee rates of characteristic and frequent values are no less than 0.90. Compared with other confidence degrees, the inferred results are more close to the true value when the confidence degree equals 0.75; therefore, it is recommended to take a confidence degree of 0.75.
-
Key words:
- load /
- characteristic value /
- Bayesian approach /
- statistical inference /
- small sample
-
中国建筑科学研究院. GB50153—2008工程结构可靠性设计统一标准[S]. 北京:中国建筑工业出版社,2009. 中国建筑科学研究院. GBJ68—84 建筑结构设计统一标准[S]. 北京:中国建筑工业出版社,1984. 冯云芬,贡金鑫,王建超. 楼面活荷载、风荷载的频遇值和准永久值的确定[J]. 工业建筑,2012,42(7): 74-78. FENG Yunfen, GONG Jinxin, WANG Jianchao. Determination of frequent value and quasi-permanent value of floor live load and wind load[J]. Industrial Construction, 2012, 42(7): 74-78. 茆诗松. 统计手册[M]. 北京:科学出版社,2003: 212-223. 周源泉. 质量可靠性增长与评定方法[M]. 北京:北京航空航天大学出版社,1997: 151-174. 黄炎生,邓浩,李涛. 考虑验证荷载的既有结构可靠度计算[J]. 华南理工大学学报:自然科学版,2008,36(10): 12-15. HUANG Yansheng, DENG Hao, LI Tao. Reliability calculation of existing structure under proof load[J]. Journal of South China University of Technology: Natural Science Edition, 2008, 36(10): 12-15. AGGARWALA R. Progressive interval censoring: some mathematical results application to inference[J]. Communications in Statistics: Theory and Methods, 2001, 30: 1921-1935. 第四机械工业部标准化研究所. 可靠性试验用表[M]. 北京:国防工业出版社,1979: 1-87. CHOPIN N, LELIÈVRE T, STOLTZ G. Free energy methods for Bayesian inference: efficient exploration of univariate Gaussian mixture posteriors[J]. Stat. Comput, 2012, 22: 897-916. TSIONAS E G. Bayesian inference for multivariate gamma distributions[J]. Statistics and Computing, 2004, 14(3): 223-233. PENG Xiuyun, YAN Zaizai. Bayesian estimation for generalized exponential distribution based on progressive type-Ⅰ interval censoring[J]. Acta Mathematicae Applicatae Sinica, 2013, 29(2): 391-402. LIN Y J, LIO Y L. Bayesian inference under progressive type-Ⅰ interval censoring[J]. Journal of Applied Statistics, 2012, 39(1): 1811-1824. 茆诗松. 贝叶斯统计[M]. 北京:中国统计出版社,1999: 75-79. 戴树森,费鹤良.可靠性试验及其统计分析(上册)[M]. 北京:国防工业出版社出版,1983: 617-628. 姚继涛. 基于不确定性推理的既有结构可靠性评定[M]. 北京:科学出版社,2011: 50-57. 中国建筑科学研究院. GB50009—2001 建筑结构荷载规范[S]. 北京:中国建筑工业出版社,2001. -
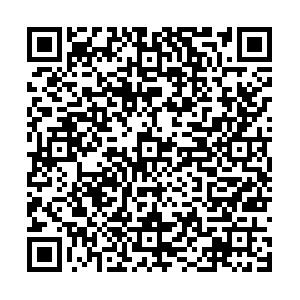
计量
- 文章访问数: 1080
- HTML全文浏览量: 60
- PDF下载量: 371
- 被引次数: 0