Image Sparse Decomposition Based on Particle Swarm Optimization with Chaotic Mutation
-
摘要: 提出了基于改进的粒子群优化(PSO)算法的匹配追踪算法,用于快速图像稀疏分解.改进的PSO算法利用尺度收缩混沌变异的精细局部搜索性能,使稀疏分解的匹配追踪算法具有良好的全局寻优能力,提高了稀疏分解在冗余字典中原子匹配的速度和准确度.用二维墨西哥草帽函数作为冗余字典的生成函数,以增强对图像边缘和轮廓的表达能力.仿真结果表明,用提出的算法实现图像稀疏分解比用遗传算法和PSO更快更有效,重建图像的视觉效果好.Abstract: A matching pursuit algorithm based on an improved particle swarm optimization(PSO) was proposed for sparse decomposition of images.The improved PSO uses the fine local search ability of the shrinking chaotic mutation to make the matching pursuit have good global search ability,improves the accuracy and increases the speed of atom matching in the redundant dictionary.The redundant dictionary employs the 2D Mexican hat function as the generating function to represent the edges and contours of images efficiently.Simulation results show that the proposed method is better than the general genetic and PSO algorithms both in speed and visual quality of the reconstructed images.
-
Key words:
- image process /
- sparse decomposition /
- matching pursuit /
- PSO /
- shrinking chaotic mutation
-
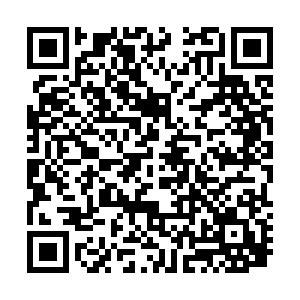
计量
- 文章访问数: 1733
- HTML全文浏览量: 97
- PDF下载量: 382
- 被引次数: 0