Data-Driven Q-Learning in Dynamic Environment
-
摘要: 针对动态环境下强化学习对未知动作的探索和已知最优动作的利用之间难以平衡的问题,提出了一种数据驱动Q-学习算法.该算法首先构建智能体的行为信息系统,通过行为信息系统知识的不确定性建立环境触发机制;依据跟踪环境变化的动态信息,触发机制自适应控制对新环境的探索,使算法对未知动作的探索和已知最优动作的利用达到平衡.用于动态环境下迷宫导航问题的仿真结果表明,该算法达到目标的平均步长比Q-学习算法、模拟退火Q-学习算法和基于探测刷新Q-学习算法缩短了7.79%~84.7%.Abstract: It is difficult for reinforcement learning to balance between the exploration of untested actions and the exploitation of known optimum actions in dynamic environment.To address this problem,a data-driven Q-learning algorithm was proposed.In this algorithm,the information system of behavior is constructed for each agent.Then the trigger mechanism of environment is build by the uncertainty of knowledge in the information system of behavior to trace the environmental change.The dynamic information of the environment is used to exploit new environment by the trigger mechanism to achieve the balance between the exploration of untested actions and the exploitation of know optimum actions.The proposed algorithm was applied to grid-world navigation tasks.The simulation results show that compared with the Q-learning,simulated annealing Q-learning(SAQ) and recency-based exploration(RBE) Q-learning algorithms,the proposed algorithm has a high learning efficiency.
-
Key words:
- reinforcement learning /
- data-driving /
- Q-learning /
- uncertainty
-
高阳,陈世福,陆鑫.强化学习研究综述[J].自动化学报,2004,30(1):86-100.GAO Yang,CHEN Shifu,LU Xin.Research on reinforcement learning technology:a review[J].Acta Automatica Sinica,2004,30(1):86-100.[2] SUITON R S,BARTO S.Reinforcement learning[M].Cambridge:MIT Press,1998.[3] ZHANG Kaifu,PAN Wei.The two facets of the exploration-exploitation dilemma[C]//Proceedings of the IEEE/WIC/ACM International Conference on Intelligent Agent Technology.Hongkong:IEEE Press,2006:371-380[4] ZHU S,DANA D H.Overcoming non-stationary in uncommunicative learning[D].New Brunswick:Rutgers University,2002.[5] WIERING M A,SCHMIDHUBER J.Efficient model based exploration[C]// Proceedings of the Fifth International Conference on Simulation of Adaptive Behavior.Zurich:MIT Press,1998:223-228.[6] KABLBLING I P,LITTMAN M L,MOORE A W.Reinforcement learning:A survey[J].J Artificial Intelligence Research,1996,4:237-285.[7] PETERS J F,HENRY C.Approximation spaces in off-policy monte carlo learning[J].Engineering Applications of Artificial Intelligence,2007,20(5):667-675.[8] VIEN N A,VIET N H,LEE S G.Heuristic search based exploration in reinforcement learning[C]//Computational and Ambient Intelligence,9th International Work-Conference on Artificial Neural Networks.Heidelberg:Springer-Verlag,4507:110-118.[9] GUO Maozu,LIU Yang,MALEC J.A new Q-learning algorithm based on the metropolis criterion[J].IEEE Transactions on Systems,Man,and Cybernetics,2004,5(34):2140-2143.[10] WANG Guoyin.Domain-oriented data-driven data mining (3DM):simulation of human knowledge understanding[C]//Web Intelligence Meets Brain Informatics.Heidelberg:Springer-Verlag,2007,4845:278-290. -
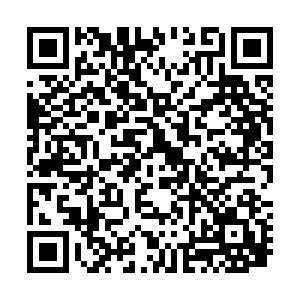
计量
- 文章访问数: 1618
- HTML全文浏览量: 111
- PDF下载量: 341
- 被引次数: 0