Fast Reduction for Large-Scale Training Data Set
-
摘要: 为了进一步减少支持向量机的训练时间,提出了一种基于类别质心的训练集缩减算法.该算法根据样本的几何分布去除训练集中大部分非支持向量.对样本规模在104数量级的数据集进行了训练实验,结果显示,在基本不损失分类精度的情况下,训练时间比直接用SMO(序贯最小优化)算法减少30%,说明该算法能有效地提高支持向量机的训练速度.Abstract: In order to cut down the time of training a large-scale data set by using SVM(support vector machine),a fast algorithm for reducing training sets was proposed based on class centroid.With this algorithm the most of non-support vectors are removed in the light of the geometrical distribution of samples.Experiments were made on several data sets at the level of 104 magnitude.The experimental results show that compared with the SMO(sequential minimal optimization) algorithm,the proposed algorithm decreases training time by 30% under the condition of ensuring the SVM’s classification accuracy to greatly improve SVM’s training speed.
-
Key words:
- support vector machine /
- class centroid /
- pattern classification
-
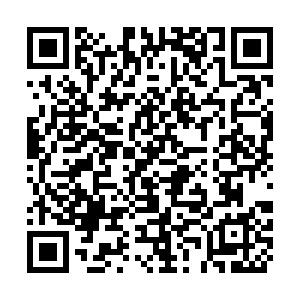
计量
- 文章访问数: 1426
- HTML全文浏览量: 66
- PDF下载量: 393
- 被引次数: 0