Calculation of Tunnel Blasting Parameters Based on Prior Knowledge and BP Neural Network
-
摘要: 为克服当前隧道爆破参数选取受人为因素影响的不足,以围岩普氏系数、隧道断面积、实际进尺和炮孔直径等为网络输入参数,以设计进尺、炸药单耗、周边孔距和掘进孔孔距等为网络输出参数,建立了含输入层、输出层和隐含层的神经网络模型,并给出了模型学习算法,提出了基于爆破先验知识的可加快模型求解收敛速度的网络学习约束条件.隧道爆破参数的实例计算结果表明,给出的网络模型及其算法能在借鉴已有爆破资料的基础上准确、快速计算爆破参数,并且获得理想的爆破效果.Abstract: To overcome the shortcoming of the parameter design of tunnel blasting,i.e.,they are selected empirically by designers,a three-layer neural network model with an input layer,an output layer and a hidden layer was constructed.The Protodikonov’s hardness coefficient,tunnel cross-section area,practical advance per round,blast-hole diameter and others are considered as the input parameters of a BP network,and the designed advance per round,powder factor,contour hole spacing,reliever-hole spacing and excavated-hole spacing as the output parameters.A algorithm for the neural network model was given,and the restrain conditions of network study were proposed on the basis of blasting prior knowledge,so solving of the model can be accelerated.The calculating results for a practical example of tunnel blasting design show that with the help of the neural network model and the algorithm,the parameters of tunnel blasting may be calculated accurately and quickly by using the existed blasting data,so an optimal blasting effect can be obtained.
-
OUCHTERLONY F.Some recent research and developments in Swedish tunnel blasting[J].Explosion and Explosives,1992,53(4):165-180.[2] SINGH S P.New trends in drilling and blasting technology[J].International Journal of Surface Mining,2000,14 (4):305-315.[3] WHITE T J,FARNFIELD R A.Computers and blasting[J].Transactions of the Institution of Mining Metallurgy,Section A:Mining Industry,1993,102:A19-A24.[4] HENDLER J,TATE A,DRUMMOND M.AI (artificial intelligence) planning systems and techniques[J].AI (Artificial Intelligence) Magazine,1990,11(2):61-77.[5] JONG Y H,LEE C I.Influence of geological conditions on the powder factor for tunnel blasting[J].International Journal of Rock Mechanics and Mining Sciences,2004,42(Sup.1):1-7.[6] JONG Y H,LEE C I.Application of neural networks to prediction of powder factor and peak particle velocity in tunnel blasting[C]//Proceedings of the Annual Conference on Explosives and Blasting Technique(Ⅱ).Las Vegas:International Society of Explosives Engineers,2002:67-76.[7] LEU Sousen,LIN S F,CHEN C K,et al.Analysis of powder factors for tunnel blasting using neural networks[J].International Journal for Blasting and Fragmentation,1998,2(4):433-448.[8] LI Xiaohong,WANG Xinfei,DONG Yongkang,et al.An expert system based on BP neural networks for pre-splitting blasting design[C]//Lecture Notes in Computer Science.Heidelberg:Springer Verlag Heidelberg,2006:1 237-1 241.[9] 靳番.神经计算智能基础原理·方法[M].成都:西南交通大学出版社,2000:123-136.[10] 张立明.人工神经网络的模型及其应用[M].上海:复旦大学出版社,1993:36-49.[11] 张继春,蔡德所,胡铁松.基于神经网络理论的岩体爆破效应预测[J].东北大学学报,1995,16(增刊):101-106.ZHANG Jichun,CAI Desuo,HU Tiesong.Rock blasting effect forecast based on neural network theory[J].Journal of Northeast University,1995,16 (Sup.):101-106.[12] 张继春.三峡工程基岩爆破振动特性的试验研究[J].爆炸与冲击,2001,21(2):131-137.ZHANG Jichun.Vibration characteristics of blasting in bed rock mass at Sanxia project[J].Explosion and Shock Waves,2001,21(2):131-137.[13] 齐景嶽,刘正雄,张儒林,等.隧道爆破现代技术[M].北京:中国铁道出版社,1999:255-264. -
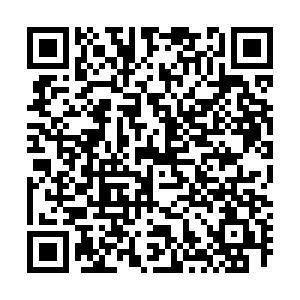
计量
- 文章访问数: 1758
- HTML全文浏览量: 82
- PDF下载量: 363
- 被引次数: 0