Compound Approach of Predicting Fault Gases Dissolved in Transformer Oil
-
摘要: 为了提高对变压器故障的预测能力,提出了灰色粗预测、自学习神经网络在线修正的复合预测法.此法是利用GM(1,1)模型初步预测油中溶解气体的浓度及变化趋势,通过分析故障气体组分之间的影响及气体浓度时间序列之间的关系确定修正参数,将初步预测结果与修正参数作为自学习BP网络的输入,从而完成预测结果的在线修正.该方法已用于实际变压器油中溶解气体的预测,结果验证了其有效性.Abstract: To improve the prediction result for transformer faults,a compound approach combining GM (1,1) model with self-learning BP-neural networks was proposed to predict fault gases dissolved in transformer oil.In this approach,the concentration and development trend of gases dissolved in transformer oil are predicted primarily using GM (1,1) model,and then the predicted results are calibrated by self-learning BP-neural networks with calibrated parameters obtained by analyzing the interaction of different types of gases and the relationship between the time sequences of gas concentrations.The proposed approach has been used in the practice of transformer fault prediction to show its validity.
-
Key words:
- transformer oil /
- prediction of fault gas /
- GM(1,1) /
- self-learning BP-neural network /
- time sequence
-
DUVAL M.New techniques for dissolved gas-in-oil analysis[J].Electrical Insulation Magazine,IEEE,2003,19 (2):6-15.[2] GUARDADO J L.A comparative study of neural network efficiency in power transformers diagnosis using dissolved gas analysis[J].IEEE Trans on Power Delivery,2001,16(4):643-647.[3] 罗运柏.用灰色模型预测变压器油中溶解气体的含量[J].中国电机工程学报,2001,21(3):65-69.LUO Yunbai.Prediction of the dissolved in power transformer oil by the grey model[J].Proceeding of CSEE,2001,21 (3):65-69.[4] 孙才新,毕为民.灰色预测参数模型新模式及其在电气绝缘故障预测中的应用[J].控制理论与应用,2003,20(5):797-801.SUN Caixin,BI Weimin.New gray prediction parameter model and its application in electrical insulation fault prediction[J].Control Theory Application,2003,20(5):797-801.[5] 王鹏.用统计学习理论预测变压器油中溶解气体浓度[J].高电压技术,2003,29(11):13-14.WANG Peng.An prediction model for dissolved gas in transformer oil based on statistical learning theory[J].High Voltage Engineering,2003,29(11):13-14[6] CHENG Shijie,ZHOU Rujing,GUAN Lin.An on-line self -learning power system stabilizer using a neural network method[J].IEEE Trans.on Power Systems,1997,12(2):926-931.[7] 付荣海,李蒙.基于PID神经网络解耦控制的变分量空调系统[J].西南交通大学学报,2005,40(1):13-17.FU Ronghai,LI Meng.Variable-air-volume air-conditioning system based on PID-ANN decoupling control technology[J].Journal of Southwest Jiaotong University,2005,40(1):13-17. -
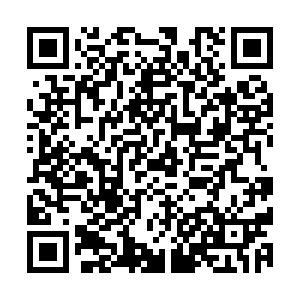
计量
- 文章访问数: 1477
- HTML全文浏览量: 73
- PDF下载量: 358
- 被引次数: 0